How to Build a Machine Learning Chatbot for Your Business

Founder & CEO at Azoft
Reading time: 24 min
Imagine a situation: Helen decided to have a seaside vacation, and she has no time to search for options – there are so many resorts! So, this task goes to a travel agency. Helen sends a message to the online chats of three tour operators. Nobody replies in the first chat. An agent from the second invites Helen to their office to check the catalogs. Finally, Pete, a smart chatbot from the third tour agency, estimates Helen’s request in the chat and offers five different trips.
Pete asked just a couple of questions. In 5 minutes, he has determined when Helen wants to have a vacation and how much money she’s ready to spend. At the same time, the chatbot promoted a tour agency and helped the business save costs on hiring additional staff to support the chat. Helen is happy and dreaming about a new bikini, while Pete the Chatbot is answering the next client’s questions. Meanwhile the tour agency owner is counting the profit.
This example shows how chatbots and chatbot apps improve client service and cut costs for the enterprise. The best thing is, chatbot building is affordable as ever, so there’s nothing that stops you from getting your own. In this article you will find an overview of the chatbot market, trends and features as well as useful tips on how to create a chatbot.
Chatbot Market Overview
The chatbot market grows bigger every day. Insider Intelligence claims that by 2024 worldwide customers will spend $142 billion via chatbots. This number becomes more impressive if we take note that in 2019 it was just $2.8 billion. Virtual assistants are on the rise because the technologies that support them (deep neural networks, machine learning) are developing fast. Advancement of AI technologies affects the chatbot industry – better bots are more attractive to business.
Source: Insider Intelligence
Chatbots have proved themselves useful to various end-user industries: banking, retail, healthcare and others. But what is so good about chatbots? The statistics answers – a lot of things! MIT technology review shows that 90% of businesses achieve faster complaint resolution using bots. Virtual assistants reduce call, chat and email inquiries by 70%. Insider Intelligence estimates that by 2023 implementing chatbots might save up to $11 million in banking, healthcare and retail sectors annually.
Chatbots are useful to customers, too. According to Saleforce, 69% of customers prefer using chatbots because they can choose the speed at which they would work with the company. 74% of users prefer communicating with chatbots in search of simple answers and 65% of customers feel more comfortable addressing an issue to a bot.
Chatbot Trends
As the chatbot market is growing rapidly and more companies consider adding chatbots to the crew, the amount of development paths and application fields for bots is increasing. You can see the overview of current bot trends on an infographic below. We will look closely at some of the hottest chatbot trends.
1. Evolution with machine learning
AI chatbots learn through human interaction fast. The training data bots collect from these interactions. The data transfers into an open source to all chatbots to use and reference during conversations. The more insights they collect, the better they become. Smooth, efficient, personalized and fast. Advancements in a machine learning field will only hasten the process.
2. Human-like touch
Tidio set up an experiment building chatbots: “robotic” and “human-like”. Over 1000 customers from the US shared their opinion on both bots. The key finding of this experiment is that people generally prefer smart bots with a pinch of personality to them. Applying natural language processing (NLP) to chatbots allows them to understand human speech patterns and present conversations with a natural flow. Lifelike bots simulating human-like interactions positively influence customer engagement.
3. Payment processing
Another hot trend is to implement chatbots as payment gateways. One of the largest online payment services Paypal has introduced a Slack chatbot that uses simple commands to transfer money between community members in 2017. Nowadays accepting, sending and requesting payments with a chatbot is more and more appealing as a customer can just type “pay for the [product name]”, cutting a lot of steps.
4. Voice recognition
Accenture report shows that customers would rather use voice-based than text-based user interface. Fortunately, in chatbot development raising conversational AI advancement caters to customer’s needs. Conversational bots prove themselves useful in travel, insurance and educational fields.
5. Social Media Chatbots
For businesses, implementation of chatbots into social media is a handy trend. Facebook messenger is starting it with their simple chatbot and other social media will more likely follow its footsteps. Rather than having an employee check and send messages from the official social media pages, it would be great to handle this responsibility to a bot that can:
- Monitor social media 24/7;
- Ensure faster response time;
- Answer to simple questions;
- Transfer leads and deals to human employees.
4 Reasons to Make Your Own Chatbot
There are a lot of reasons for you to consider creating your own chatbot. Bots have massive advantages for business and the customers. Let’s review some of the most popular reasons to create a chatbot.
1. Optimize workspace: for customers and yourself
Gartner claims that by 2022 around 70% of office workers will include a bot into their daily routine. The ability of bots to process and understand verbal commands provides great help in cutting down tedious tasks to a simple phrase. Asking bot to schedule a meeting, remind of important events or fetch old documents saves time and effort.
Picture below illustrates an example of how the chatbots can automate your working processes and save your time.
Jane is trying to fetch a PDF for project A stored somewhere on her desktop cloud storage application Box. To do this, Jane has to follow a 6-step process. By using a chatbot, she only makes a single verbal request.
2. Improve customer service
Custom chatbots have great advantages when it comes to communication with your clients. Implementing a chatbot in your technical support team you will be able to:
- Provide 24/7 customer support;
- Automate typical request processing:
- Increase sales;
- Save money on customer services team;
- Give your company a friendly face, presenting it to the customers.
3. Acquire customer data
Evolving, chatbots are collecting data from user input. You can use these insights to personalize customer experience for your website and mobile application, showing up products and information they are interested in.
4. Keep products in stock
In connection with IoT (Internet of things) chatbots can notify warehouse employees to stock up on a certain product, schedule and announce the shipments.
Chatbot Features
Chatbot functionality heavily depends on a chatbot’s purpose. Picking and choosing your chatbot capabilities keep in mind your customers intentions, goals and expectations from the conversation. You might even consider writing user stories. Would they want to sign up for an appointment? Find advice on using your product? Modern chatbots are highly customizable, but share essential features:
Ability to learn from experience and mistakes
Whatever the purpose of the chatbot is, it should be able to accumulate knowledge and use it for your and your customer’s advantage. Conversations with customers should refine your chatbot’s ability to imitate human speech and problem-solving ability. Allowing users to leave feedback in case of chatbot mistakes might help in bot learning how to complete complex tasks. Progressive profiling allows you to collect data efficiently and create personalized user experience.
More than just text
Conversations may be unpredictable and sometimes text messaging is not enough to describe a feature or a product. Enabling rich messaging – allowing chatbot to interact using photos, videos and audio will definitely improve customer service. Adding voice command recognition and speech synthesizers would be a great idea too. Another way for chatbot to evolve is to improve accessibility for clients that might have complications related to visual and hearing impairments.
Multiplatform communication and integration
The abundance of devices and platforms associated with them requires chatbots to be available everywhere. Make sure to tune your chatbot for seamless experience within browsers, mobile applications, messaging platforms and elsewhere. Chatbots may also be integrated into your company’s infrastructure tohandle software updates, automate payments and more.
Secure
With chatbots that collect customer data and process payments, it is vital to make them secure and compliant to the best cybersecurity practices. Regulations like Data Protection Act (DPA) and Data Protection Ordinance should apply. Besides that, make sure that your chatbot gives the customers accurate and correct information. If the bot makes a mistake that leads to client’s financial loss, the company will be liable.
The Chatbot Development Steps
Let’s look at chatbot development steps based on our experience of creating a machine learning chatbot for our HR department
The Azoft HR department often faces a situation when an employee asks a question. Despite the information being available on the corporate web portal, some employees might prefer communicating their problems to HR directly rather than searching the website. These problems can pile up in a day, leaving no time for HR officers to do their actual job.
Therefore we decided to build a chatbot that can determine the needs of Azoft employees and answer their questions. We implemented an R&D study and made a machine learning chatbot for the Azoft HR department. Our virtual assistant for HR is based on a recurrent neural network.
1. Developing an architecture
Before the project began we made two key requirements for our custom chatbot:
- The Chatbot has to answer customer inquiries.
- The Chatbot has to engage in dialogue similar to a live conversation to give the impression of “being there”.
First, we created an architecture where the chatbot could answer inquiries from users but couldn’t ask counter questions. We got a simple algorithm of natural language processing or user’s phrase processing:
- Convert a user’s phrase from text to a numeric vector.
- Process a numeric vector with a User Intent Classifier.
- Choose a phrase to meet a user’s inquiry from the list of prepared phrases that coincide with a User Intent
The simplest chatbot architecture
2. Improve customer service
Custom chatbots have great advantages when it comes to communication with your clients. Implementing a chatbot in your technical support team you will be able to:
- Provide 24/7 customer support;
- Automate typical request processing:
- Increase sales;
- Save money on customer services team;
- Give your company a friendly face, presenting it to the customers.
3. Acquire customer data
Evolving, chatbots are collecting data from user input. You can use these insights to personalize customer experience for your website and mobile application, showing up products and information they are interested in.
4. Keep products in stock
In connection with IoT (Internet of things) chatbots can notify warehouse employees to stock up on a certain product, schedule and announce the shipments.
Chatbot Features
Chatbot functionality heavily depends on a chatbot’s purpose. Picking and choosing your chatbot capabilities keep in mind your customers intentions, goals and expectations from the conversation. You might even consider writing user stories. Would they want to sign up for an appointment? Find advice on using your product? Modern chatbots are highly customizable, but share essential features:
Ability to learn from experience and mistakes
Whatever the purpose of the chatbot is, it should be able to accumulate knowledge and use it for your and your customer’s advantage. Conversations with customers should refine your chatbot’s ability to imitate human speech and problem-solving ability. Allowing users to leave feedback in case of chatbot mistakes might help in bot learning how to complete complex tasks. Progressive profiling allows you to collect data efficiently and create personalized user experience.
More than just text
Conversations may be unpredictable and sometimes text messaging is not enough to describe a feature or a product. Enabling rich messaging – allowing chatbot to interact using photos, videos and audio will definitely improve customer service. Adding voice command recognition and speech synthesizers would be a great idea too. Another way for chatbot to evolve is to improve accessibility for clients that might have complications related to visual and hearing impairments.
Multiplatform communication and integration
The abundance of devices and platforms associated with them requires chatbots to be available everywhere. Make sure to tune your chatbot for seamless experience within browsers, mobile applications, messaging platforms and elsewhere. Chatbots may also be integrated into your company’s infrastructure tohandle software updates, automate payments and more.
Secure
With chatbots that collect customer data and process payments, it is vital to make them secure and compliant to the best cybersecurity practices. Regulations like Data Protection Act (DPA) and Data Protection Ordinance should apply. Besides that, make sure that your chatbot gives the customers accurate and correct information. If the bot makes a mistake that leads to client’s financial loss, the company will be liable.
The Chatbot Development Steps
Let’s look at chatbot development steps based on our experience of creating a machine learning chatbot for our HR department.
The Azoft HR department often faces a situation when an employee asks a question. Despite the information being available on the corporate web portal, some employees might prefer communicating their problems to HR directly rather than searching the website. These problems can pile up in a day, leaving no time for HR officers to do their actual job.
Therefore we decided to build a chatbot that can determine the needs of Azoft employees and answer their questions. We implemented an R&D study and made a machine learning chatbot for the Azoft HR department. Our virtual assistant for HR is based on a recurrent neural network.
1. Developing an architecture
Before the project began we made two key requirements for our custom chatbot:
- The Chatbot has to answer customer inquiries.
- The Chatbot has to engage in dialogue similar to a live conversation to give the impression of “being there”.
First, we created an architecture where the chatbot could answer inquiries from users but couldn’t ask counter questions. We got a simple algorithm of natural language processing or user’s phrase processing:
- Convert a user’s phrase from text to a numeric vector.
- Process a numeric vector with a User Intent Classifier.
- Choose a phrase to meet a user’s inquiry from the list of prepared phrases that coincide with a User Intent
An example of how we converted a sentence to a numeric vector using the bag-of-words algorithmThis vector includes all the words that can be met in a user’s phrase.
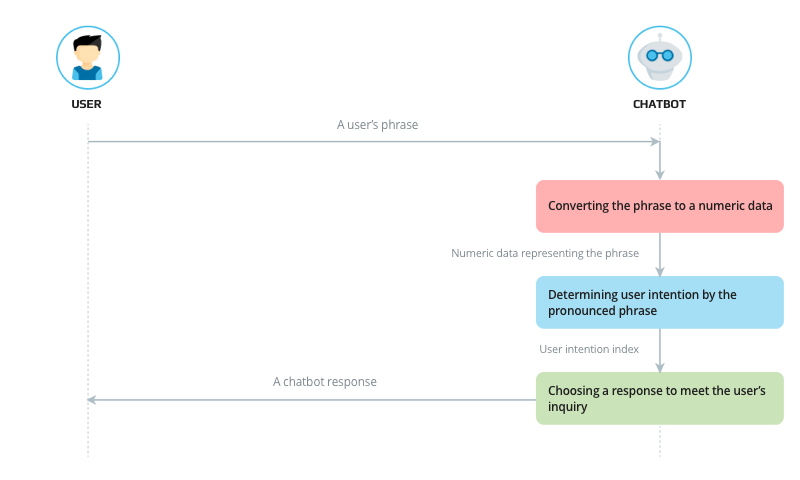
The final scheme of communication between a user and chatbot based on the bag-of-words
To classify the users intentions we used a DecisionTreeClassifier from the sci-kit-learn library.
The bag-of-words algorithm turned out to be quite precise and handled even situations where unfamiliar words were used.
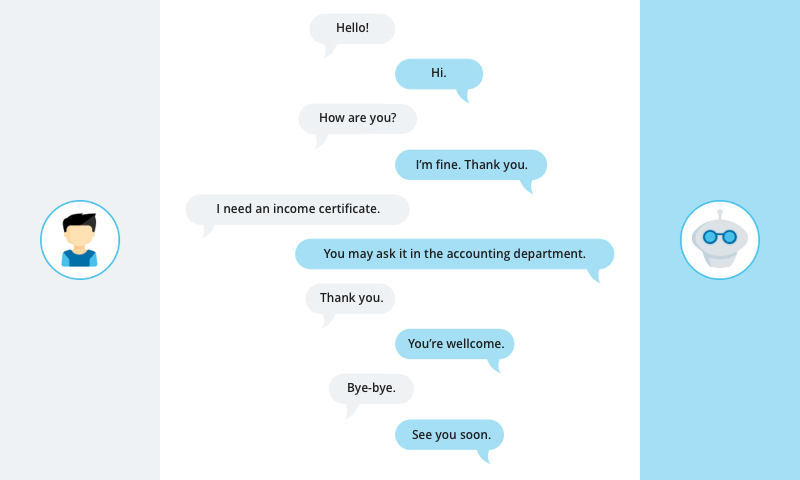
A conversation with a smart chatbot based on the bag-of-words algorithm
3. Refine the chatbot architecture to more complex
A “bag-of-words” chatbot could easily answer questions but it didn’t create a live conversation flow. It couldn’t ask questions. For this reason, we decided to let our chat pal ask questions. Thus we started to change the chatbot architecture.
First, we created a new entity UserIntent. It keeps information about user intention, a set of phrases that can express this intention, and actions that can be done in response to the user inquiry.
Another entity we had was Action. It helps determine what actions a chatbot has to take to respond to the user inquiry. This change allowed the chatbot not only to answer a user with a predefined phrase but also take actions that help to create a new phrase in response to the user’s question.
For example, an employee asked the chatbot to find a coworker’s phone number. Then the algorithm turns to the base of contacts of all the user colleagues to find a required phone number. It’s necessary to implement all the mentioned actions to fulfill this user’s inquiry.
Besides Action and UserIntent, we added an entity DataEntity that expresses all the data needed to ask a user for.
When a chatbot doesn’t know the user’s intention, it waits for the entered phrase. A user says something and the chatbot tries to recognize the user’s intention from the phrase. If the chatbot succeeds, it can take an action to fulfill the user inquiry. If there is enough data, the chatbot takes an action and “forgets” a user’s question as it’s already answered. If there is not enough data, the chatbot returns to the user with a request to give additional data. So this is what a conversation between the chatbot and a user could look like.
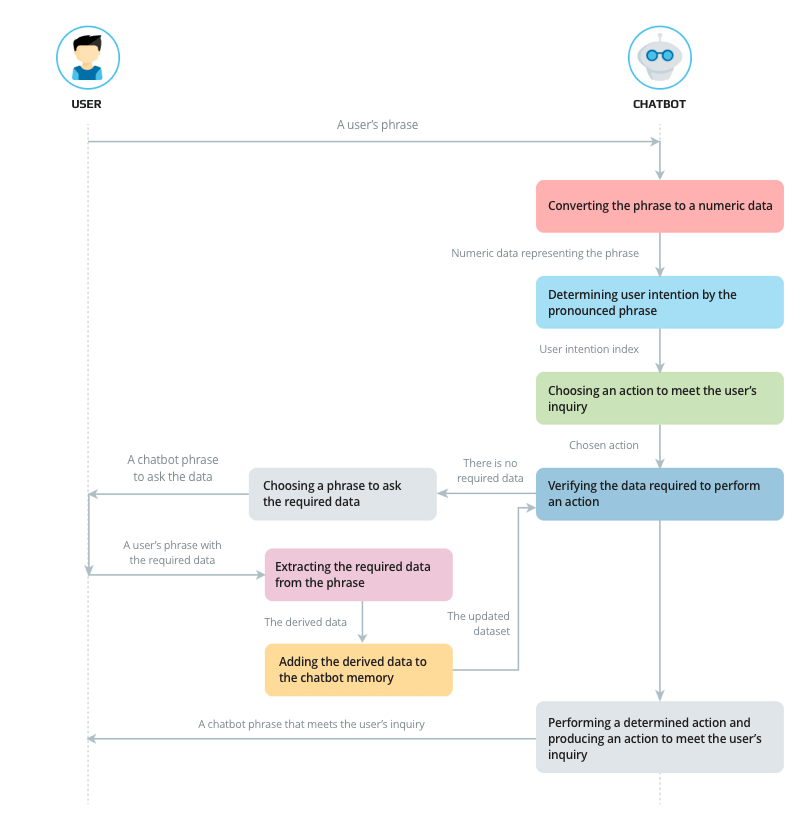
Architecture of interaction between a user and a chatbot
4. Changed the model in favor of neural networks
The decision tree model based on the bag-of-words algorithm showed pretty good results for recognizing written text. But when we increased the number of user intents, the chatbot vocabulary grew. This led to the growth of elements in the bag-of-words vectors derived from the user phrase processing. As a result, we had to spend more and more time training the chatbot. This didn’t work for us and we decided to train a neural network to determine the users intention.
We applied an already trained model word2vec from the open Facebook AI repository to convert phrases into numeric vectors. This type of model produces a corresponding numeric vector for every word.
At this stage, we did experiments with a neural network of MLP (multi-layered perceptron) architecture. A numeric vector of the user’s phrase formed all of the numeric vectors sum of all the words in a phrase. However, the MLP model was unsteady to the new words that appeared in questions but weren’t mentioned during training.
We settled on the neural network model with a bi-directional LSTM layer because we’ve successfully used it for other tasks with nlp machine learning.
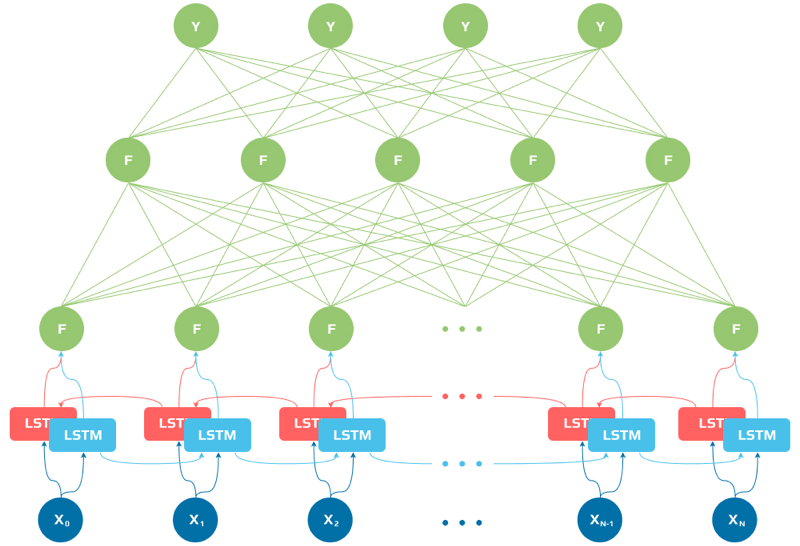
Architecture of the neural network model with a bi-directional LSTM layer
A machine learning chatbot model based on the neural network recognized user intentions perfectly. The neural network analyzed every word separately considering all the neighboring words and only then decided what a user wants.
When we changed the chatbot architecture and used another model of the neural network to classify phrases based on the user’s intention, we got a smart chatbot. Our AI chatbot can answer user questions and ask follow-up questions as well.
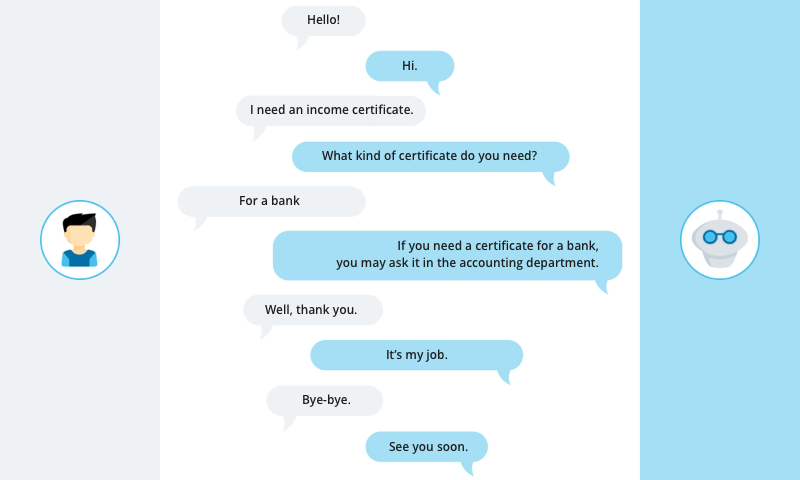
Example of conversation between a user and neural network-based chatbot
5. The Outcome
We developed a smart chatbot on the basis of a neural network that determines what a user wants just on their phrase. Our chatbot demonstrated 99,9% accuracy in understanding natural language during a conversation.
Our study showed that the word2vec algorithm can be applied to get numeric vectors. Unlike the bag-of-words algorithm which widens in proportion to the number of words in the chatbot’s vocabulary.
We plan to add new user intentions to the chatbot base of knowledge and to implement data search in the user’s phrases.
How Much Does It Cost to Develop a Chatbot?
The development process is unique for every project and chatbot creation is no different. To estimate the cost of building a chatbot you will have to define the scope of your product. A simple bot can be done in a few days for free using one of many bot development platforms. A more refined and sophisticated bot with expanded functionality will consume more time and money. Such bots will have to go throught the full development cycle, including analytics, design, code writing, testing and quality assurance. Bugfixes and further refinement of the bot will also bring additional expenses.
Create Bots with Azoft
A chatbot can become a powerful tool to increase company revenue, but bot building platforms often are not enough to create a complex multi-functional chatbot. If you are interested in chatbot development, contact us at info@azoft.com to acquire help from an experienced software development company.
Comments